Michael Lewis’ book and the adapted film, Moneyball, provide valuable advice for people involved with the selection and retention of employees. However, judging from some reviews, there is confusion about the message in Moneyball. I describe the problem and the Moneyball solutions here. These solutions are valuable for personnel decisions in any large organization.
Problem: Finding the right person for a given position is a highly complex task, yet experts believe that their experience allows them to solve such a complicated problem using intuition alone. Unfortunately, no one can learn from experience to determine what will work in complex, uncertain situations. A summary of decades of empirical evidence led to the “Seer-sucker Theory” for forecasting (Armstrong 1980): “No matter how much evidence exists that seers do not exist, suckers will pay for the existence of seers.” Evidence since that time, such as Tetlock’s 2005 book, Expert Political Judgment, has provided overwhelming support.
Despite the evidence, most people believe they are immune from the Seer-sucker Theory. They find reasons to rationalize their dependence on unsubstantiated instinct and think that their situations are different. Note how the baseball scouts—who epitomized baseball’s contemporary thinking—were convinced that Billy Beane, the Oakland As’ general manager, was wrong when he disregarded opinions offered from gut feelings and years of experience. According to Lewis, many people associated with professional baseball, not just the scouts, thought Billy Beane was nuts—and a danger to baseball—by relying only on statistics.
Solutions: Billy Beane used two key procedures to select and retain his baseball players: the first relates to developing statistical models, and the second to ensuring the models are properly used. Professor Paul Meehl at the University of Minnesota, summarizing his and other research, had long before recommended evidence-based procedures for dealing with these aspects of personnel selection. Meehl published his conclusions in his 1954 book, Clinical vs. Statistical Prediction. Additional research supported Meehl’s findings (see the review by Grove, et al, 2000). The findings have been widely cited and have been taught in universities for about half a century.
The first procedure from Meehl’s research is based on findings that statistical models do better than unaided judgments for personnel decisions. Billy Beane analyzed the data on players’ performance and used the models’ outputs to select players. He understood that opinions should be used only as inputs to a model. In other words, do not revise the models’ recommendations based on opinions. Meehl used an example to explain this principle. It went something like this:
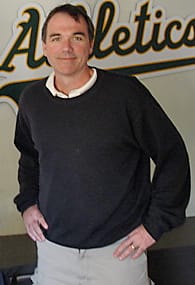
Billy Beane
You go to the checkout lane of a supermarket and pile up your purchases. It would be insane to say, “It looks like $178.50 to me.” You perform the calculations. And once the calculations are done, you do not say, “Well, in my opinion the groceries were a bit cheaper, so let’s reduce it by $8.00.” You adhere to the calculations.
Interestingly, despite the overwhelming evidence, few organizations use models to predict job performance. Most continue to use their judgments about how people would perform. The more they think about it, the more confident they become in their opinions.
To illustrate this point, I’ll use examples from my own experience. In the early 1970s, I was flying from Denver to Philadelphia. Some fit, young men were on the flight. Wondering who they were, I turned to the person sitting next to me to see if he knew. He did. His name was Ed Snider, and he owned the Philadelphia Flyers, the hockey team whose players were on this flight. Before we were out of Colorado, I realized that this was my big chance. I would convince him to employ me to select hockey players by using predictive models. Sports writers would learn about me. Other teams would then flock to my door. I would become rich and famous.
So, after a suitable interval, I asked, “Tell me, Ed, how do you select your players?”
He told me that his managers had recently been using a model to make the decisions. He said that the Dallas Cowboys used a model, and they were known for making good draft picks. Originally, he was the only one in the Flyers’ organization who thought it would work. His managers resisted, but after a two-year experiment, they agreed that the new approach was better. The players that the model selected did better than the ones that the managers selected.
Life gave me a second chance at fame. In 1979, on a visit to my friend Paul Westhead, I suggested developing models to make personnel decisions for the Los Angeles Lakers. Although it was his first year coaching the Lakers, Paul, well known for his creativity, loved the idea. I proposed a modest amount of funding (little more than petty cash for the Lakers), but the owner, Jerry Buss, turned it down. As it happened, the Lakers won the championship in that 1979-80 season without me. (I think Magic Johnson helped.)
In performance-based models we have a method that clearly works and would lead to enormous gains. After more than half a century, it has been used for personnel selections by a few sports teams and perhaps two universities. Billy Beane, with help from Michael Lewis to communicate the message, changed this. Many baseball, basketball, soccer, hockey and football teams now use performance models. For example, in the first part of the National Basketball Association’s 2009-10 season, the 15 teams with at least one full-time statistician on their staff won 59 percent of their games, while the 15 teams with no statisticians won only 41 percent (David Biderman, Wall Street Journal, March 12, 2010).
Still, I doubt that many business firms use this procedure.
Moneyball makes developing a model sound complex. It is not for dummies, but then many people can master it. The tool you need is on your computer—the regression program on a Microsoft Excel spreadsheet. Regression analysis was developed in the 1800s. Since then, the big advance is that it is now much cheaper to collect and analyze data thanks to computers. Of course, there are many ways to misuse regression analysis (see Armstrong 2012). However, if you keep things simple, ignore statistical significance and use prior knowledge as an input to your model, you are likely to substantially improve your decisions in personnel selection.
I, along with two colleagues, am now working on a method that is simpler, more effective and less likely to be misused compared with regression. This method, developed by Benjamin Franklin, predates regression analysis. We call it the “index method.”
Assume that you have to select one of a number of candidates. Our advice is to list all of the criteria that are known to be important and assign a point for each criterion on which each candidate does well (in some cases it may help to weight the variables). Add the points across criteria for each candidate, and then select the candidate with the highest score. We have recently been comparing predictions from this index method against predictions based on regression models in predicting U.S. presidential elections. Index models are accurate and they are more informative than regression models. The primary expense will be to develop a list of variables that have been shown to be valid for performance for the job in question. Summaries of this research can be found on PollyVote.com.
Fortunately, an enormous amount of research has been published on personnel selection. However, few personnel consultants use the literature. For example, when ranking the importance of variables (e.g., cognitive skills), personnel selectors’ conclusions are virtually unrelated to the evidence-based rankings (e.g., Ahlburg 1992).
So what was Meehl’s second procedure? You should not meet job candidates until you decide to make them an offer. This procedure seems preposterous to people. Nevertheless, it leads to better decisions. If you do not follow this procedure, you might override your model’s recommendations and thus harm decision-making. People are influenced by many biases, some of which are subconscious. For example height, weight, gender and looks are often used even when they are not relevant to job performance.
Some organizations have applied Meehl’s second procedure. For example, Goldin and Rouse (2000), in their study of symphonic orchestra auditions, found that when the screening committee does not see the applicant (they play behind a screen) female applicants were much more likely to pass this stage of the recruitment process. Billy Beane used this procedure; he did not even watch his team’s baseball games.
Business firms have more to benefit than sports teams, because in sports, the advantage begins to disappear when other teams also use models. In contrast, every large organization would continue to benefit from Meehl’s procedures for personnel selection. Applicants would also benefit from better decisions. In addition to more accurately forecasting which candidate will be most effective, the models are less expensive. The use of models can also eliminate bias due to variables that are not related to performance, such as gender, race, religion or political beliefs.
Meehl’s findings also apply beyond personnel selections. In reviewing the evidence on predictions about people, he concluded in 1956 that “it almost looks as if the first rule to follow in trying to predict the subsequent course of a student’s or patient’s behavior is to carefully avoid talking to him, and the second rule is to avoid thinking about him.”
Change occurs slowly. Professor Meehl passed away in 2003 after a long and illustrious career. Michael Lewis published Moneyball that same year.
Fortunately for you, when you adopt these procedures for selecting people—such as your next CEO—few of your competitors will do so. How do I know? I learned this from Winston Churchill: “Men occasionally stumble across the truth, but most of them pick themselves up and hurry off as if nothing had happened.”
(Editor’s note: This blog is based on a paper to be published in Foresight: The International Journal of Applied Forecasting.)
References
Ahlburg, Dennis A. (1992). “Predicting the job performance of managers: What do the experts know?” International Journal of Forecasting, 7, 467-472.
Armstrong, J. Scott (1980), “The seer-sucker theory: The value of experts in forecasting,” Technology Review, 83 (June/July), 18-24.
Armstrong, J. S. (2012), “Illusions in regression analysis,” International Journal of Forecasting. 28 [Forthcoming; available at http://papers.ssrn.com/sol3/papers.cfm?abstract_id=1969740]
Goldin, C. & C. Rouse (2000), “Orchestrating impartiality: The effect of ‘blind’ auditions on female musicians,” American Economic Review, 90 (4), 715-741.
Grove, W.M. et al. (2000), “Clinical versus mechanical prediction: A meta-analysis,” Psychological Assessment, 12, 19–30.