Growing up in Mumbai, Bhakti Vithalani WG06 had always been struck by the extreme income disparities among the residents of this coastal Indian city. She also developed a fascination for technology and its power to transform societies. But these twin themes would not come together until Vithalani’s college years, where she studied computer science. It was in this academic setting that an idea began to bud: Why not use technology to deliver learning at scale to anyone who wants gainful employment?
Vithalani went on to pursue a Wharton MBA and outlined a more concrete business plan in her admissions essay. However, it was not until she worked at McKinsey that she decided to act on this plan, after co-authoring a report stating that only 20 percent of Indian engineering graduates were deemed employable. That was when “the pin dropped,” Vithalani says. “There’s no shortage of people. It was actually a shortage of skill sets.” This was the genesis of her startup, BigSpring.
Today, Vithalani is the CEO of BigSpring, a lifelong skilling platform that enables employers to measure ROI from learning. It uses AI to recommend skills for users to learn and vet the content they generate, at scale. The content that users generate helps demonstrate their proficiency. The startup doesn’t just award learners certificates and assume that they are equipped for work; it also measures their proficiency. BigSpring’s customers include companies such as Google, Uber, Tata Steel, and Sotheby’s.
Vithalani, CTO Arun Nagarajan, and director of operations Yin Yin C06 W06 WG06 discussed BigSpring’s vision as a disruptor and force for good with Wharton Magazine. The following is an edited version of the conversation.
Wharton Magazine: What will jobs of the future look like? Could you discuss the key trends that could impact the future of work?
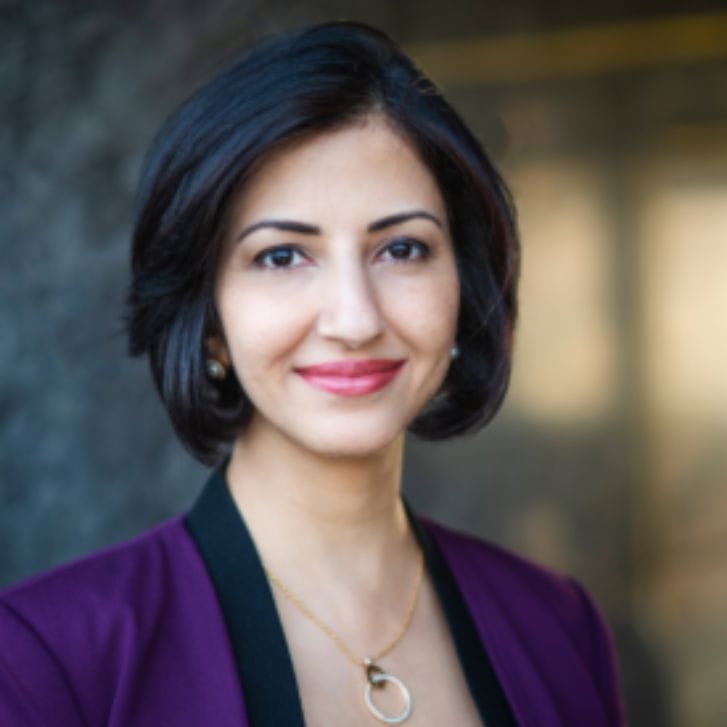
Bhakti Vithalani WG06
Bhakti Vithalani: There has been a perpetual debate about the jobs of the future. This is literally economics. If the economy is advancing, there is a need for upskilling and there will always be a skills gap. Our approach and framework are built around the idea that rather than predicting the jobs of the future, we create a platform and the machinery where no matter what that pace of change is, no matter how unpredictable and volatile it is, we can have the mechanism to upskill people on whatever skills are needed.
There are other foundational and evergreen skills — such as critical-thinking, design thinking, diversity and inclusion, mental health, and resilience — that are relevant across job functions and industries.
Then there are more dynamic areas. Macro trends such as digitization and the demand for digital tools literacy, for instance. Automation is going to displace hundreds of millions of workers. It will not necessarily make them unemployable, but they need to be upskilled to move on to better and higher value-added activities. So that is definitely a shift. If you look at COVID and what it has done to retail and hospitality — those sectors are getting heavily digitized. The world has become cashless. Many things are going to be more delivery-focused and remote than before.
Wharton Magazine: Arun, how do AI and analytics play into this?
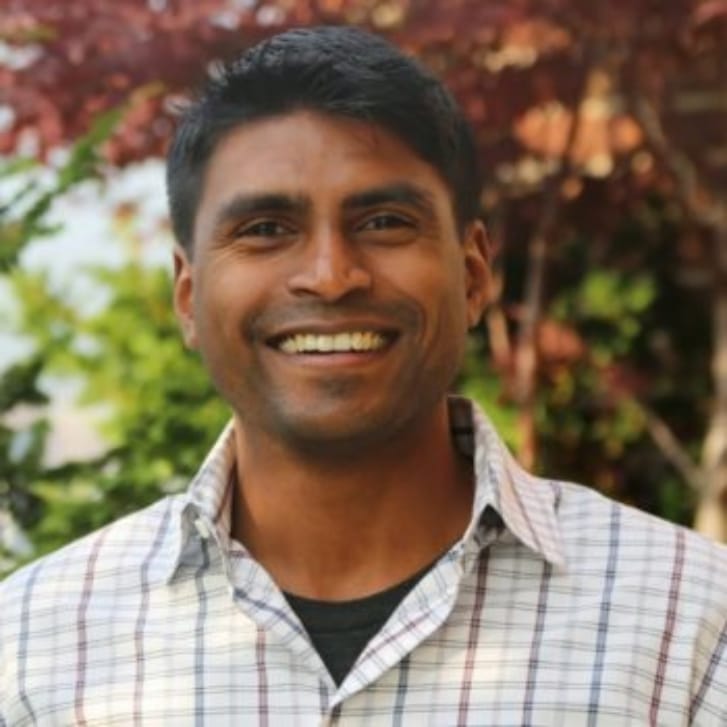
Arun Nagarajan
Arun Nagarajan: On AI and analytics, it’s now reached a point where it just becomes embedded in a lot of these products, and a lot of what we want to achieve can’t be done without it. That is one of the reasons why we are not inventing new analytics models. We are not machine-learning researchers, and we are not figuring out how to use GPUs to do better analytics.
It is more about, “How do we use practical applications of state-of-the-art technologies?” Given that preface, the way we use AI comes down to two big buckets. One is the content and skills we recommend to people. We have a catalog of universal skills — the 500 that matter, and then the employers themselves will have skills unique to their organization
You end up with potentially hundreds of immediately available skills to be learned on our platform that may be suitable for hundreds of employees. So how might you do the first pass of mapping what skills apply to which people? This part will be a bit more rules-based, not necessarily AI-based, such as factoring in that you are in a certain department, or you’ve only been with the company for three months.
Let’s say you, the employee, are now progressing with the training. If you’re not transferring teams and you’ve been in your position for a couple of years, maybe some new skills have become more important than the skills you have now. That is where we have started to experiment with AI, which recommends and prioritizes what you should do next, and some of the things you should explore. That is one area of AI implementation.
The second area where we are using AI is in assistive technology to help us parse user-generated content. When I, as a learner, am now asked to demonstrate my skills proficiency, usually you would record a video or audio of yourself or take a screenshot and get feedback. But you could upload objectionable or sensitive content, or you may completely miss the mark and record something that’s low quality or fuzzy. AI is useful in moderating such content. In the process, you are also saving a human multiple hours of back and forth with the learner.
Using AI, we also can do some speech analysis. Let us say it’s a public speaking skill being learned, and the person is not pausing enough, or they are monotonic. We play with AI models where we can detect such things and provide private feedback.
Vithalani: Our repository and our source of deriving relevance is the real world. Some 70 percent of content on BigSpring comes from our enterprise customers. The recommendations we make to people are based on what enterprises are demanding, which is extremely in flux, and gets updated frequently.
Wharton Magazine: Could you offer an example of a company that is using what you have developed, and what you have learned so far?
Vithalani: Google came to us about a year ago and said, “Google Ads has 500 feature updates every year.” They have an ecosystem of hundreds of thousands or even millions of practitioners at agencies and vendors that need to be upskilled. It could take more than 12 months to upskill the ecosystem. They needed a platform to rapidly deploy skills, at pace with product innovation, to ensure product adoption.
The first step was helping them to rapidly produce and deploy content. We have this YouTube-style approach where we had Google subject matter experts record training videos for our platform. We started with two features, and now we are expanding that library. The first generation of coaches were Google subject matter experts, and now coaching is propagating across the ecosystem. The first-generation learners are becoming the second-generation coaches, and so on.
We are committed to peer learning on the platform for learning efficacy and engagement but we’re also solving for scale. We are collecting and evolving a dataset for our AI algorithms to be trained on. If one person had been able to work with 10 learners, they can now work with 100 or even 1,000. And if those 1,000 learners were getting best practices from their immediate peers, they can now get best practices from the world. It becomes scalable and generative — every person on BigSpring becomes a force multiplier.
We envision a world where there is a learning ecosystem, with an enterprise that sits in the middle of it generating a dynamic pool of market-relevant skill sets through the content. Then, each person in the ecosystem is helping to upskill and support a few others in their peer group. Aided by AI, the scale is unlimited.
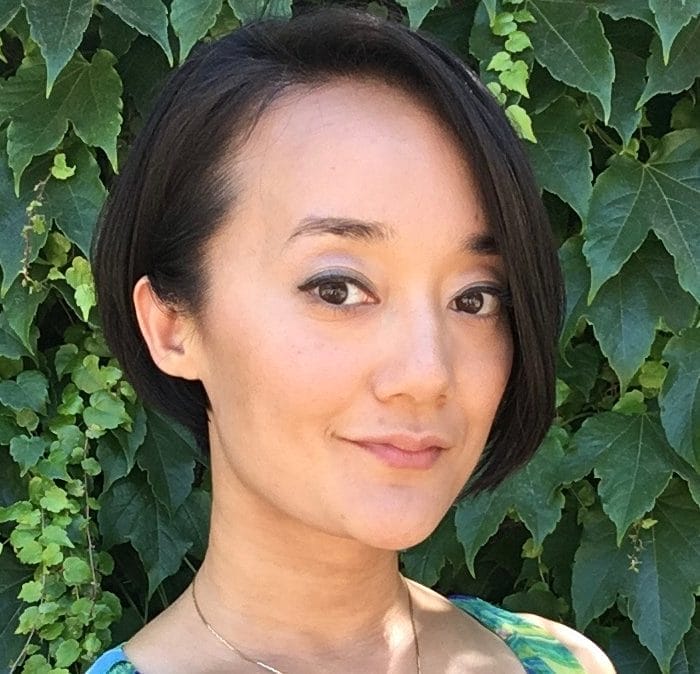
Yin Yin C06 W06 WG06
Yin Yin: There are a few different pieces we want to help Google operationalize to turn out content at the real pace of its innovation. One piece we have already shown, just through a few months of working with the Google team, is they can now turn around content that used to take months to develop down to two weeks, and we are going to shrink that down even more.
Google experts themselves are creating content like YouTube stars, and we enable them through the BigSpring Creator Academy and with playbooks and equipment. The content is all bite-sized; it’s not so heavy. But rather, here’s a little bit, and you can finish it quickly and at your own pace.
Ultimately, we are shooting to make the content as easy and accessible as possible, and for it to be applicable. That is the main point of upskilling these workers.
Mukul Pandya is the founding editor in chief and executive director of Knowledge@Wharton. He retired recently from Penn after running K@W for more than 22 years.