In their new book, Superforecasting: The Art and Science of Prediction, Wharton professor Philip Tetlock and co-author Dan Gardner share the results of decades of research into futurism. The following is based on an interview between Tetlock and Knowledge@Wharton.
By Philip Tetlock and Dan Gardner
Imagine you’re a big-shot pundit. What incentive would you have to submit to a forecasting tournament in which you had to play on a level playing field against ordinary human beings? The answer is: not much. The best possible outcome you could obtain is to tie it. You’re expected to win. But there’s a good chance, our research suggests, that you’re not going to win.
In our early work, which goes back into the mid-1980s, we used the metaphor of the dart-throwing chimp to capture a baseline for performance—which is, if you had a system that was just generating forecasts by chance, how well would you do relative to that?
That actually is a baseline that some people can’t beat. For a lot of reasons. Sometimes, the environment is just hopelessly difficult. If you were trying to bet on a roulette wheel in Las Vegas, you’re not going to be able to do any better than a dart-throwing chimp. But people sometimes fail to beat the dart-throwing chimp even in environments where there are predictable regularities that could be picked up if they were being astute enough.
You don’t want to be too hard on people because there’s a lot of irreducible uncertainty in some environments. It’s very difficult to bring down the uncertainty below a certain point. It’s unfair to portray people as being dumb, in some sense, if they’re failing to do something that’s impossible. Of course, we don’t know what’s impossible until we try. Until we try in earnest. You don’t discover how good you can become in a particular forecasting environment until you run competitive forecasting tournaments. You plug in your best techniques for maximizing accuracy. You see how good you can get.
That’s essentially what we did in the forecasting tournaments with the U.S. government, one sponsored by the intelligence community, the Intelligence Advanced Research Projects Agency, or IARPA. These are forecasting tournaments that were run between 2011 and 2015 involving tens of thousands of forecasters trying to predict about 500 questions posed by the intelligence community. We found that some people could do quite a bit better than the dart-throwing chimp, and they could beat some more demanding baselines as well.
We recruited forecasters by advertising through professional societies and through blogs. A number of high-profile bloggers helped us to recruit forecasters, people like Tyler Cowen and Nate Silver. We were able to gather initially a group of several thousand, and we were able to build on that in subsequent years.
I have to be careful about making big generalizations about how good or bad people are as forecasters. As I mentioned before, you can make people look really bad if you want to. You can pose intractably difficult questions. Or you can make people look really good. You can pose questions that aren’t all that hard. So you want to be wary of research that does cherry-picking.
What we were looking for was a process of generating questions that wasn’t rigged one way or the other. The method we came up with was generating questions through the U.S. intelligence community. They were questions that people inside the U.S. intelligence community felt would be of national security interest and relevance and reasonably representative of the types of tasks that intelligence analysts are asked to do. The questions asked people to see out into the future several months, occasionally a bit longer, occasionally shorter. We scored the accuracy of their judgments over time.
We didn’t have people make judgments one way or the other. It wasn’t yes or no. We had people make judgments on what’s called a probability scale ranging from zero to one. We carefully computed accuracy over time. We identified some people who are really good at making these judgments—we called them “superforecasters”—and they were later assembled into teams. They dominated the tournament over the next four years. But we did a number of other experiments and looked for techniques that could be used to improve accuracy, and we found some.
Ask people in the political world, “Who has good judgment?” The answer typically is, “People who think like me.” Liberals tend to think that liberals have good judgment and good forecasting judgment, and conservatives tend to think that they are better at it. It turns out that good forecasting accuracy is not very closely associated with ideology. There’s a slight tendency for people who are superforecasters to be more moderate and less ideological, but there are lots of superforecasters who have strong opinions. What distinguishes superforecasters is their ability to put aside their opinions, at least temporarily, and just focus on accuracy. That’s a very demanding exercise for people.
Eventually, you’re going to reach a point where you’re not going to get any better because, as I mentioned, the environment itself has some degree of irreducible uncertainty. So no matter how good you are, you’re probably not going to do a very good job predicting what the value of Google is going to be next week on Nasdaq. There are some things that are very difficult to do. It’s not clear that even using superforecasters is going to let you make appreciable headway on that.
But there are many things that are quite doable that we previously didn’t think were doable, and there’s a lot of room for improving the accuracy and probability judgments on those things. Those are things like predicting whether international conflicts are going to escalate or de-escalate, whether certain treaties are going to be signed or approved by legislatures, or whether Greece is going to leave the eurozone—many problems that have relevance to financial markets and to business decisions, where there is potential to improve probability judgment and where people typically don’t do that. People typically rely on vague verbiage forecasts. You’ve heard people say, “Well, I think it’s possible. This could happen. This might happen. It’s likely.” Those terms are not all that informative.
If I say that something could happen—for example, Greece could leave the eurozone by the end of 2017—what does that mean? It could mean there’s a probability of 1 percent or a probability of 99 percent. Or we could be hit by an asteroid tomorrow. Asking people to make crude, quantitative judgments, which become progressively more refined over time, is a very good way to both keep score and get better at it.
Fox and the Hedgehog
In our book Superforecasting, we refer to the famous fox-hedgehog metaphor, drawn out of a surviving fragment of poetry from the Greek warrior poet Archilochus, 2,500 years ago. Scholars have puzzled over it for centuries. It runs something like this, and of course, I don’t know ancient Greek, so I’m taking on faith that this is what it actually says: “The fox knows many things, but the hedgehog knows one big thing.”
Think of hedgehogs in debates over political and economic issues as people who have a big ideological vision. The writer Tom Friedman might be animated by a vision of, say, globalization: the world is flat. Libertarians are animated by the vision that there are free market solutions for the vast majority of problems that beset us. There are people on the left who see the need for major state intervention to address various inequities. There are environmentalists who think we’re on the cusp of an apocalypse of some sort. So you have people who are animated by a vision, and their forecasts are informed largely by that vision.
Whereas the foxes tend to be more eclectic. They kind of pick and choose their ideas from a variety of schools of thought. They might be a little bit environmentalist and a little bit libertarian, or they might be a little bit socialist and a little bit hawkish on certain national security issues. They blend things in unusual ways, and they are harder to classify politically.
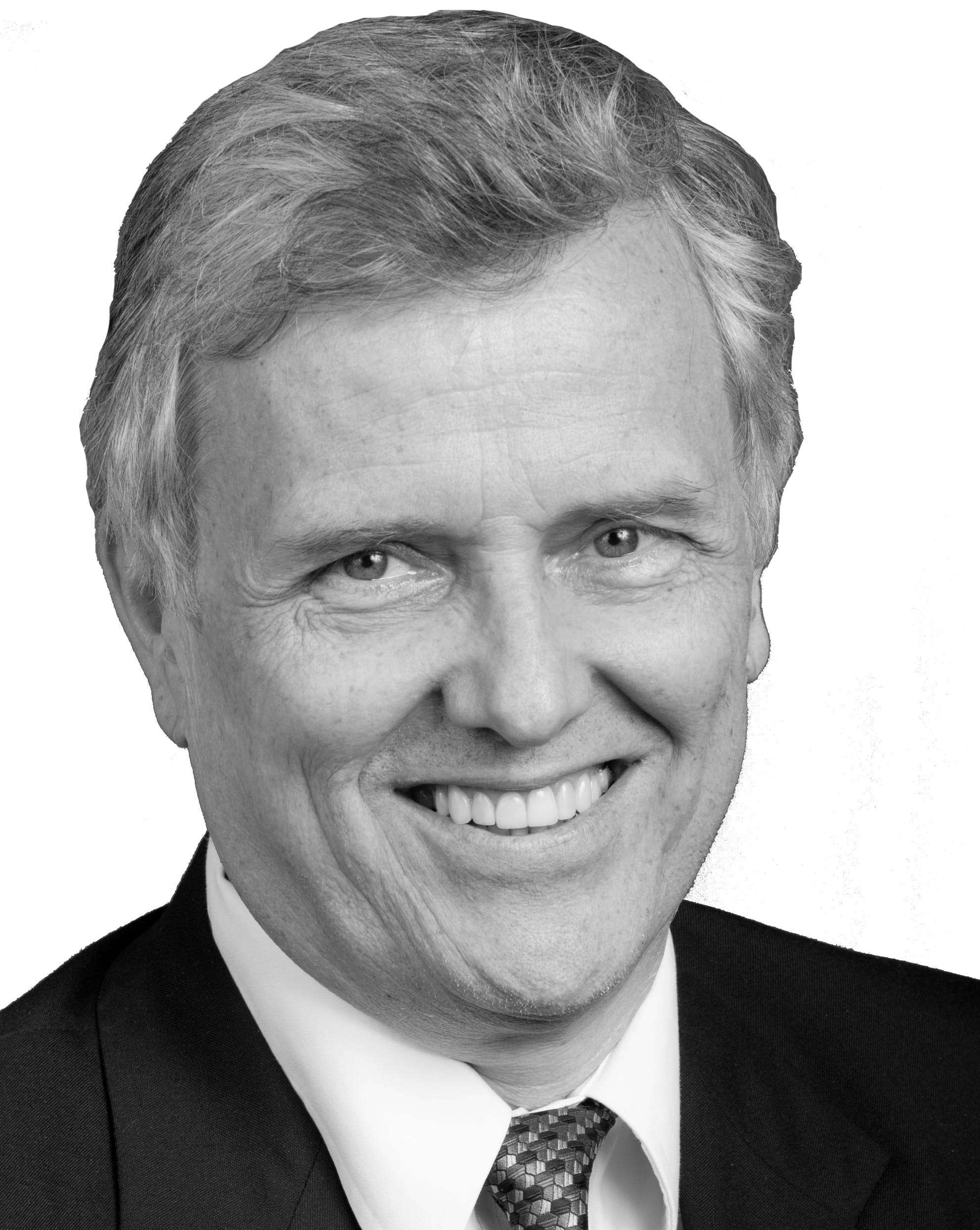
Philip Tetlock, Management
In our early work, we found that the foxes who were more eclectic in their style of thinking were better forecasters than the hedgehogs. In later work, we found something similar. We found that people who scored high on psychological measures of active open-mindedness and need for cognition tended to do quite a bit better as forecasters.
Now, imagine you are a producer for a major television show, and you have a choice between someone who’s going to come on the air and tell you something decisive and bold and interesting—the eurozone is going to collapse in the next two years, or the Chinese economy is going to melt down or there’s going to be a jihadi coup in Saudi Arabia. He’s got a big, interesting story to tell, and the person knows quite a bit and can mobilize a lot of reasons to support the doom-and-gloom prediction. The person is charismatic and forceful.
Contrast that with someone who comes on and says, “Well, on the one hand there’s some danger the eurozone is going to melt down. But on the other hand there are these countervailing forces. On balance, probably nothing dramatic is going to happen in the next year or so, but it’s possible that this could work.” Who makes for better television? To ask the question is to answer it.
There is a preference for hedgehogs in part because hedgehogs generate better sound bites. People who generate better sound bites generate better media ratings, and that is what gets people promoted in the media business. So there is a bit of a perverse inverse relationship between having the skills that go into being a good forecaster and having the skills that go into being an effective media presence.
The Question Generator
Let’s use the example of Tom Friedman versus someone like Bill Flack. Friedman is, of course, a famous New York Times columnist, a Pulitzer Prize winner who is a regular at Davos, and the White House and circulates in networks of power. Flack is an anonymous, retired hydrologist in Nebraska who also is a superforecaster. We know a huge amount about Flack’s forecasting track record because he answered a very large number of questions in the course of our tournament and demonstrated he could do so effectively. But we know virtually nothing about Friedman’s forecasting track record, notwithstanding that he’s written a great deal over the last 35 years and that he’s a powerful analyst and a writer who has done many things very well. But there’s no way to reconstruct with any degree of certainty how good a forecaster he is. Friedman has detractors, he has admirers. His admirers might say, “Well, he was right that it was a bad idea to expand NATO eastward because it would provoke nationalist backlash in Russia.” Or, “He was wrong about Iraq because he supported the 2003 invasion.”
We did a careful analysis of Friedman’s columns, however, and one of the things we noticed is even though it’s very difficult to discern whether he’s a good forecaster, going back after the fact, it is possible to detect some really good questions. He’s a pretty darn good question-generator. We’ve actually begun to insert some of his ideas for questions. They tend to be rather open ended. We’ve managed to translate some of them into future forecasting tournaments.
But there’s tension between being a super question generator and a superforecaster. Here’s an example: Before the 2003 Iraq invasion, Friedman wrote a column on Iraq in which he posed the following question, which really cut to the essence of a key issue in deciding whether to go into Iraq: Is Iraq the way it is today because Saddam Hussein is the way he is, or is Saddam Hussein the way he is because Iraq is the way it is?
It’s the old chicken-and-egg question. What would happen if you took away Saddam Hussein? Would the country disintegrate into a war of all against all? Or would it move toward a Jeffersonian liberal democracy in the next 15 or 20 years? Friedman didn’t know the answer to that question. Many people think he made a big mistake in supporting the invasion of Iraq in 2003, but he was shrewd enough to pose the right question. If we’d been running forecasting tournaments in late 2002, early 2003, that would have been something we would have wanted very much to include in that exercise.
The right way to think about Friedman and Flack is that they are complementary. Friedman’s greatest contribution to forecasting tournaments may well be his perspicacity in generating incisive questions. He may be a good forecaster, too, but we just don’t know that yet.
Sometimes it’s also a matter of reframing the questions. In our book Superforecasting, we conducted an interview with David Ferrucci. When he was an IBM scientist, he was responsible for developing a famous computer program known as Watson, which defeated the best human Jeopardy players. We asked him about his views about the human/ machine forecasting. One line of questioning was particularly interesting. It was very clear to him that it would be possible for a system like Watson to answer the following question reasonably readily: Which two Russian leaders traded jobs in the last five years? For that question, Watson could search its historical database and figure it out. What if we reframe the question as: Will those same Russian leaders change jobs in the next five years? Would Watson have any capacity to answer a question like that? Ferrucci’s answer was: no. Our next question was: How difficult would it be to reconfigure Watson so that it could answer a question like that? His answer: massively difficult. Think about what would be required to do the sorts of things that superforecasters collectively do—the amount of informed guesswork that goes into constructing a reasonable forecast—it is difficult to imagine artificial intelligence systems doing that in the near term.
Getting Down to It
A lot of people spend quite a bit of money on advice about the future that probably isn’t worth the amount of money they are spending on it. They have no way of knowing that because they have no way of knowing the track record of the people whose advice they are seeking.

Michael Platt
The best example is probably in the domain of finance where a lot of money changes hands and is directed to people who claim to have some ability to predict the course of financial markets. That is an extraordinarily difficult thing to do. I’m not saying it’s impossible or that nobody can do it any better than a dart-throwing chimp, but it’s a very difficult thing to do. If people were more skeptical about the people to whom they turn for advice about possible futures, I think finance would be a case in point. But, I think, more generally people should be very skeptical of the pundits they read and the claims that politicians and other people make about the future as well. It’s very common for people to make bold claims about the future and offer no evidence for their track records. It’s almost universal.
It comes down to human psychology. We take our cues about whether somebody knows what he or she is talking about from how confident he or she seems to be. That’s a problem, and it suggests that people need to think a little bit more carefully when they make appraisals of competence and not rely quite as heavily as they do on what we call the “confidence heuristic.” It is true that confidence is somewhat correlated with accuracy, but it’s also possible for manipulative human beings to use that heuristic and turn us into money pumps.
The answer is to learn for ourselves what makes a good prediction. And that answer? It’s a focus on the questions themselves.
Philip Tetlock’s research interests include “responsibility” and “assessing good judgment”—social phenomena not commonly discussed in academia. But his career has been crossing boundaries for decades. His titles alone speak to that diversity: Leonore Annenberg University Professor in Democracy and Citizenship, Professor of Management, and Professor of Psychology. His research in judgment led to the creation of forecasting tournaments, bringing together 284 expert forecasters and 28,000 predictions. Other tournaments have followed, producing forecasts about geopolitics for the intelligence community. His research eventually led to his latest book.
Published in the Spring/Summer 2016 issue of Wharton Magazine.