There are many great moments in Michael Lewis’ Moneyball, but one of my favorites involves an exchange between Dan Feinstein, the Oakland A’s video coordinator, and newly acquired outfielder John Mabry.
Mabry and teammate Scott Hatteberg are commiserating over how difficult it is to prepare for a game against Seattle Mariners ace Jamie Moyer, a soft tosser who manages to confound hitters with an 82 mph fastball and unhittable outside pitches that are too slow to resist swinging for the fences on.
In the face of this seemingly insoluble puzzle, Feinstein has some obvious advice: “Don’t swing, John.”
To which Mabry responds with the classic ad hominem: “Feiny, have you ever faced a major league pitcher?”
To me this incident encapsulates the fundamental tension between those who actually work in professional sports and the superfans who are trying to get in—if you want to say something meaningful about the game, you better have actually played.
I’ll grudgingly admit there’s probably something to this argument. Because if you’re picking names out of a hat to find a Major League Baseball general manager, you probably will do better by picking someone who has played the game than a random fan who never got beyond teeball.
Thankfully, those who own sports franchises don’t have to just pick names out of a hat. This is where the old boys’ club of former players bumps into a serious problem. By hiring former players to run teams, owners are treating a specific kind of experience as an implicit proxy for knowledge, wisdom and intelligence. All of these might come with experience, but there are no guarantees. Moreover, as the Oakland A’s and other teams have shown, there are other ways of acquiring those same qualities in a GM.
Lewis may not have expressed it this way (but I don’t think he’d disagree with me either), but until the Oakland A’s took the sport in a different direction, Major League Baseball had made a fetish out of playing experience, turning it into an end in itself rather than understanding the proxy that it was.
As I watch professional hockey teams hire droves of their own superfans to adapt the sports analytics thinking developed in baseball to a new context, it’s worth sounding a note of caution: Many within the hockey analytics community have their own fetishes—chief among them repeatability, sustainability and an affinity for large data sets.
Repeatability, sustainability and large data sets are fundamental to any serious analysis of hockey, meaning they shouldn’t be challenged lightly.
So let me explain.
As any fan knows, the outcome of a game comes down to a combination of ability and luck. If two teams play a lot of games, the better team wins more often, but in any single game (or a short playoff series), upsets happen.
The trick for people who do what I do is to find numbers that tell us whether a team won because it’s actually good or was just lucky.
Hence the obsession with repeatability and sustainability. Skills that can be replicated from game to game lead to consistent winning. Lucky bounces don’t.
But many hockey analysts run into problems once they start to disconnect the particular measure they’re looking at from the broader question: Is this team actually good or bound to fail?
Take the example of PDO, a meaningless acronym that’s simply the sum of a team’s overall shooting percentage (the number of shots that result in goals) and its save percentage (the percentage of opponents’ shots that its goalie prevents from going in the net).
Consensus in the hockey analytics community is that a “high” PDO can’t be sustained, meaning over the long term a team’s PDO will regress to a mean level and that team will start losing games.
There’s some lively debate around what a “normal” PDO is. Often people will simply assume a team’s shooters couldn’t all be that much better than the league average, and therefore, any PDO above 100 should be looked at with suspicion.
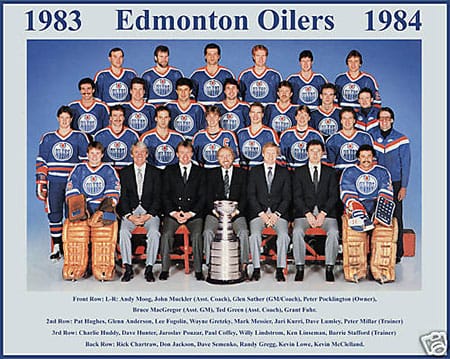
One of the best teams in professional hockey history sustained extraordinarily high PDOs for an entire season. Explain that. Photo credit: wikia.com.
Others are a bit looser, tolerating something above 100 for really strong teams but setting some not entirely defined ceiling at which they become suspicious.
On average, the analysts may be right, but there’s a problem with the logic: Two of the greatest teams in NHL history—the 1983-84 Edmonton Oilers and the New York Islanders that squared off against them in the Stanley Cup final—both got outshot and sustained extraordinarily high PDOs for an entire season. (See my piece “Is There Luck in Hockey” for more explanation.)
As my colleague Phil Curry recently discovered, if you’re trying to predict future performance in the middle of the season, PDO is a statistically relevant variable. In other words, teams with higher PDOs continue to win—either because above- average team shooting percentages and save percentages are sustainable after all, or else the PDO regresses and other abilities emerge.
Regardless, it appears that PDO is—in some cases at least—reflective of skill rather than luck.
Last of all, there’s the problem of large data sets. Like anybody who works with data, I generally have a bias toward looking at as much data as I can find. But sometimes less really is more.
If you’re adding observations that are only tangentially related to the hypothesis you’re considering, all you’re doing is introducing noise that obscures a relationship that exists in some subset of the data.
Take the example of shooting a hockey puck at a net.
Last season, I was curious to see whether some top scorers did a better job of hitting the net than others. I looked at 2.5 seasons of data for 27 top goal scorers (to be precise, it was the top 25 for 2.5 years running that I had looked at in an article about six weeks earlier, plus Sidney Crosby, who it seemed a shame to exclude simply because of some ill-timed injuries).
As it turned out, some guys consistently hit the net, while others didn’t.
I dug a bit further and what I found was really interesting (although not perhaps entirely surprising): those who shot from “in-close” did a better job of hitting the net than those who shot from far away. (For more, read “The Relationship Between Shooting Distance and Shot Percentage on Net.”)
What happened next was a little odd.
A blogger with whom I occasionally bounced ideas (hired this summer by an NHL team) came back and concluded, based on data he had seen for the entire NHL over half a season, that there was no relationship between shooting distance and hitting the net.
According to a study he had read (and agreed with), if you were trying to guess the likelihood of whether or not a player would get a shot on net rather than miss or have the shot blocked before it got there, it didn’t matter whether that player shot from far away or in close. To use the language of hockey analytics, hitting the net from any distance was a matter of luck or randomness rather than a repeatable skill.
That struck me as a little odd since it was the exact opposite of what both intuition and my own data suggested, but I thought about it some more and what became clear was that all the additional data had introduced a great deal of noise into the equation.
I had only looked at elite goal scorers—players who fired the puck a lot and had a chance of actually scoring when they did.
By looking at the entire league over a short time frame, the other sample included a bunch of nobodies—guys who were happy merely to have the puck on their stick from time to time and who didn’t shoot often or necessarily pick spots when they did.
The hypothesis I was testing—is there a reason why some elite scorers hit the net more often than others—required an examination of elite scorers only. Adding the other 95 percent or so of the league didn’t help answer that question. Rather, it obscured the answer by introducing a whole bunch of noise.
What I found strange was the absolute faith this particular analyst had in his data despite the fact that it flew in the face of what seemed pretty obvious: All things being equal, shooting the puck from further away increases the chances of missing or having someone block the puck on its journey.
Three of the most-heralded analytics hires in the NHL this offseason were in Edmonton (as of this writing, 29th in the standings among 30 teams), New Jersey (24th but possibly improving) and Toronto (27th and in freefall). For selfish reasons, I was rooting for all those teams to succeed, and to be fair, they all have legacy issues that need more time to resolve themselves before anyone can say whether or not the analytics are “working.”
But what’s increasingly clear in hockey, as in all areas that are being transformed by big data, is that data don’t apply themselves. Concepts like repeatability, sustainability and sample size are useful, but only if you understand how and when to apply them. To do that, it takes a good qualitative understanding of whatever subject you’re studying (in my case hockey), constant attention to whether the data are relevant to the hypothesis you’re testing, and the creativity and analytical chops to interpret whatever your scatter plot, histogram or regression model might be telling you.
When undertaking that exercise, fetishes and preconceptions aren’t helpful. At worst, they’re as dangerous as the old guard’s stubborn adherence to prioritizing playing experience for its own sake.