In The Signal and the Noise, Nate Silver provides a fascinating survey of humanity’s love affair with the art and science of prediction. One of the historical figures Silver discusses is 19th century French astronomer and mathematician Pierre-Simon Laplace, who posited that people would be able to make perfect predictions about the natural world if they had three things going for them: (i) perfect information about the location of every particle; (ii) perfect knowledge of natural laws; and (iii) sufficient computational power.
In North America’s four major team sports, conditions (i) and (iii) have essentially been met. We may not know what is happening at a subatomic level when a change-up is thrown on a wet and windy May day at Coors field, but the technology that allows teams to measure, track, record and replay every meaningful mechanical characteristic of that ball is in wide use. And we’re certainly not short of computing power to allow us to crunch equations and validate our theories with the most massive data set we can put together.
Unfortunately, condition (ii) is a tougher nut to crack.
A decade or so ago, the debate between stats geeks and baseball’s old-timers raged over this very question. Were baseball’s “natural laws” really so easy to understand that a bunch of nerds on laptops could run regressions and spit out predictions better than guys who had been steeped in years of experience?
Today, the debate is settled with a “yes—to a point.” All Major League Baseball teams use advanced statistical methods, but they haven’t ditched their front offices, coaching staffs and scouts either.
Baseball is a highly static (some might say boring) sport in which outcomes are defined and individual contributions are relatively easy to understand. Each team starts with 27 outs, and one of four things happens: a hit, a walk, an out or an error. Putting aside errors, which are relatively rare, what happens after a pitch leaves the mound is almost entirely about a pitcher and a batter. Sure, there are great defensive plays, and of course random luck enters the conversation, but in the overwhelming majority of cases, a hit would be a hit against any team, and in a long season, where a player who avoids injury can expect no less than 486 plate appearances, luck tends to even out.
Baseball also benefits from having an enormous data set, compiled by several decades of statisticians and casual fans occupying themselves in many of the game’s “quieter” moments by meticulously logging every play.
In my sport, hockey, things are more complicated.
The game doesn’t have a huge following outside of Canada, is played at a high speed and is difficult for a novice to follow.
My 3–year-old may be bored by baseball, but in hockey she is routinely asking if her favorite player, Penguins forward Chris Kunitz, is “on the ice or resting?”—and where the puck is.
What would be more confusing for her is the question of whether Kunitz is actually a star or rather just a middling player we talk about simply because Sidney Crosby, arguably the world’s best, feeds him the puck.
Another challenge with hockey is that outcomes are not fixed. Whether a game is high or low scoring depends very much on the system each team employs, and as my colleague IJay Palansky noted, it’s not clear that either a defensive or wide-open system is inherently better, let alone the many variations within each of those categories.
Moreover, the goal in baseball remains the same from beginning to end: get on base when on offense; prevent the other guy from getting on base when on defense.
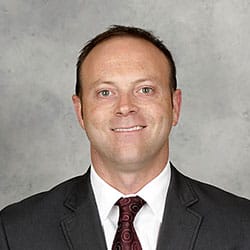
Stan Bowman, vice president and general manager of the NHL’s Chicago Blackhawks, credits analytics for his team’s success.
In hockey, a team plays a different style depending on whether it’s ahead, behind or tied, and how much time is left on the clock.
The NHL was also a six-team affair until 1967, and the data that people in my line of work like to use aren’t available on a game-by-game basis going back even that far.
Last of all, while walks and hits happen relatively frequently in a 162-game baseball season, goals don’t happen very often in a hockey game, and the season is only 82 games long.
As a result, hockey is going through the same philosophical battle that baseball went through in the 1990s and early 2000s. Indeed, when my colleagues and I were talking about launching the Department of Hockey Analytics this past fall, a number of my close friends who are rabid hockey fans expressed constructive skepticism.
Nevertheless, advanced statistics are slowly gaining acceptance in the mainstream hockey world. Newly appointed Philadelphia Flyers general manager Ron Hextall publicly stated his intention to build an analytics team (rumored to have already been hired). The New Jersey Devils, recently purchased by two Wharton alumni, are currently hiring a director of analytics.
Chicago Blackhawks general manager Stan Bowman publicly credited analytics as his secret weapon. The Blackhawks have won two of the past four Stanley Cups and made the conference finals for the fourth time this year out of the past six. That’s a remarkable feat in a 30-team league with a salary cap.
As my colleague Phil Curry wrote recently, this year’s NHL playoffs looked to be full of upsets based on regular season standings; however, from the perspective of advanced statistics, the NHL’s results were not surprising.
Since launching in January, the Department of Hockey Analytics has been riding this wave and having a lot of fun doing it.
Nevertheless, the hockey-isn’t-baseball crowd does have a point. The nature of the game makes it difficult to tease out individual contributions and it’s folly to assume there will be a single “holy grail” statistic that will tell us everything we need to know. It would be equally foolish, however, to pretend—as some teams appear to be doing—that no insight can be gained from looking at the data.
As in baseball, the future will be about combining a qualitative understanding of the game with an understanding of the math and its limitations—and being able to explain those insights to the teams and fans.
As a former agent for hockey players who has spent thousands of hours in rinks now working with a former player and a team of economists, that makes this a pretty exciting time for me.